Data Day Texas 2025 - Key Takeaways

The data professionals are not okay. We may put on a good face and talk about how excited we are that AI is going to change the world, but behind that mask of professional enthusiasm, we are anxious. Nowhere was this more evident than at the Data Day Texas Town Hall, hosted by Joe Reis and Matt Housley for the 3rd year running. Are executives going to replace us with AI? We knew that AI would likely impact other industries, but will it also reduce job opportunities in the industry responsible for building AI systems? AI is supposed to improve our productivity, to be a tool that we use to do bigger and better things, not a cost-saving mechanism for corporate executives trying to show that this quarter’s numbers are better than expected… right? We talk about how Copilot is just like a super productive intern - but what about the actual interns? If we stop hiring juniors and only hire seniors and supplement them with code generators, what happens in 10 years when suddenly there are no seniors to hire, because they couldn’t get a junior role? Even before that, are students learning the right skills to get hired into an entry-level role? Computer Science programs have been in crisis for a while now, curriculums more and more outdated with every passing year, and students are starting to question the value of a 4-year degree when they can only learn the skills jobs are asking for in bootcamps. Are we, the data professionals who have seized on AI trends as a way to justify our budgets (after all, your AI system is only as good as your data!), in fact building ourselves out of our jobs (not because we should really be replaced, but because the AI is cheaper and seems to be good enough), pulling up the career ladder behind us (why hire juniors if they won’t be productive enough for years), and undercutting the entire higher education system without anything to replace it?
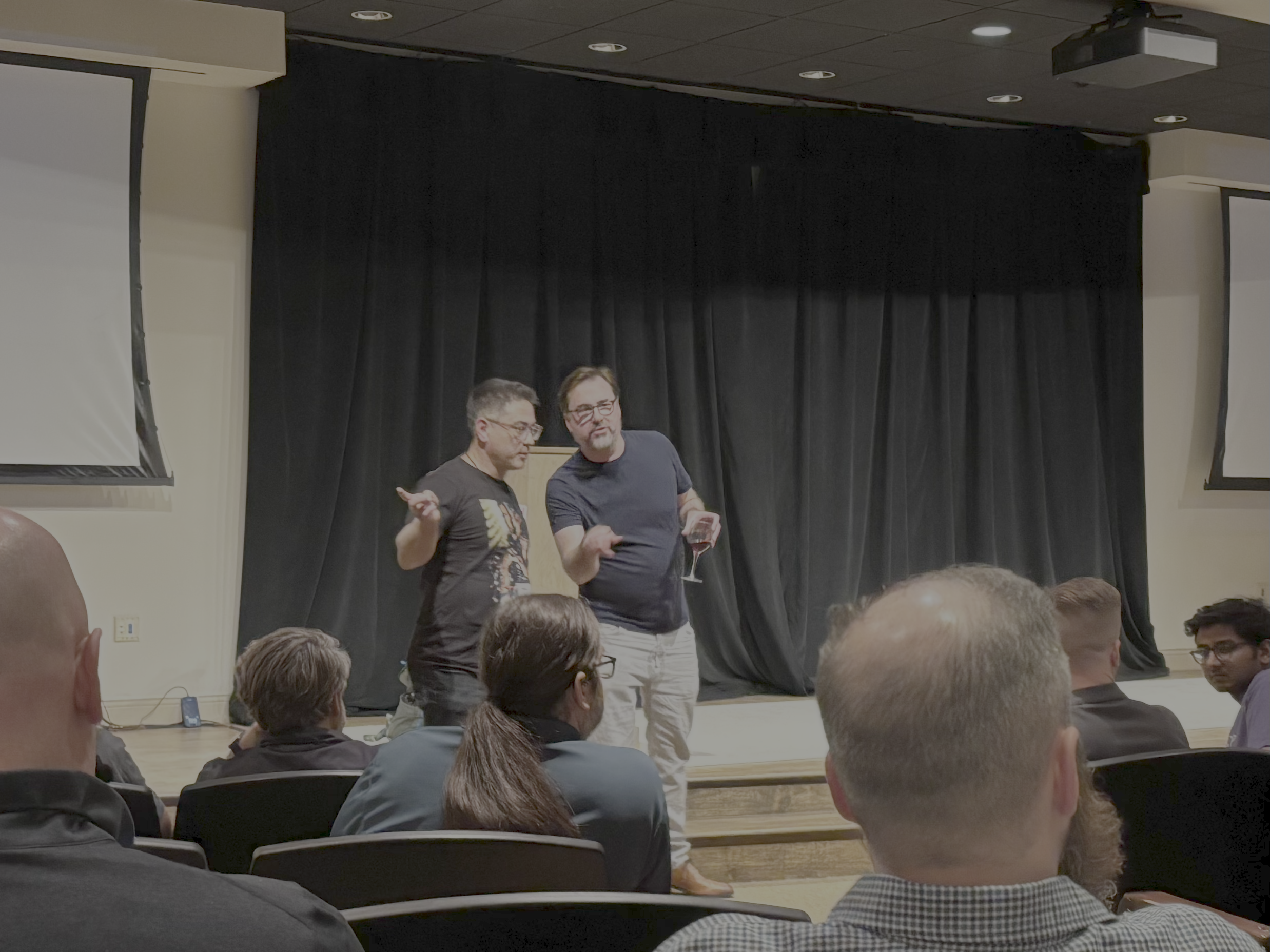
Yeah, we’re anxious - all of these concerns and more were raised in the Town Hall. Of course, there were also the AI optimists, who debated the AI doomers with cheerful counterpoints that a utopia of sky-high productivity and dramatically more free time was just around the corner (though I’m reminded that Keynes thought we’d be working 15 hour work weeks by now), that AI would solve far more problems than it caused (hopefully it starts with the climate crisis, and codes itself into being carbon neutral), and that human ingenuity and adaptability would always prevail, and jobs will just look different rather than going away (though this tends to work across generations, individuals still get left behind). As Ole pointed out the next day during discussions, it’s ironic that the nation with one of the worst social safety nets is charging ahead with AI so determinedly while other developed nations where you can lose your job without worrying you may also lose your life have been dragging their feet on AI - one would think that paradigm should be flipped.
While the Town Hall gave DDTX attendees a chance to give voice to these anxieties in a massive group Data Therapy session (classic Joe Reis), the actual talks offered guidance on how to navigate this next year - the skills data professionals need to focus on, new concepts we need to learn and integrate into our practices, and some old technologies made new again that will be especially relevant in building AI that will actually live up to our hopes (and not our fears). I found the Town Hall comforting - to know that I wasn’t the only one anxious about these things - and the talks inspiring, charting a path forward that I am actually excited for. My experience at Data Day Texas was heavily biased by my interests, given that there were 6 talks happening at once throughout the day (and then 3 discussions at once the next day) and nothing was recorded, but I got a chance to learn about at least some of the talks I couldn’t see live through conversations with the other attendees. (There was one discussion that was recorded due to being live streamed - a town hall style session hosted by Joe Reis and the Super Data Brothers on AI in BI, available here).
There were 3 key themes tying the talks together that I noticed:
- Learnings from Library & Information Science (and, context is still king)
- “Human” (soft) skills help us connect with the business (and help them)
- Data Mesh is a journey, and we’re now in the land of Data Products
Learnings from Library & Information Science (and, context is still king)
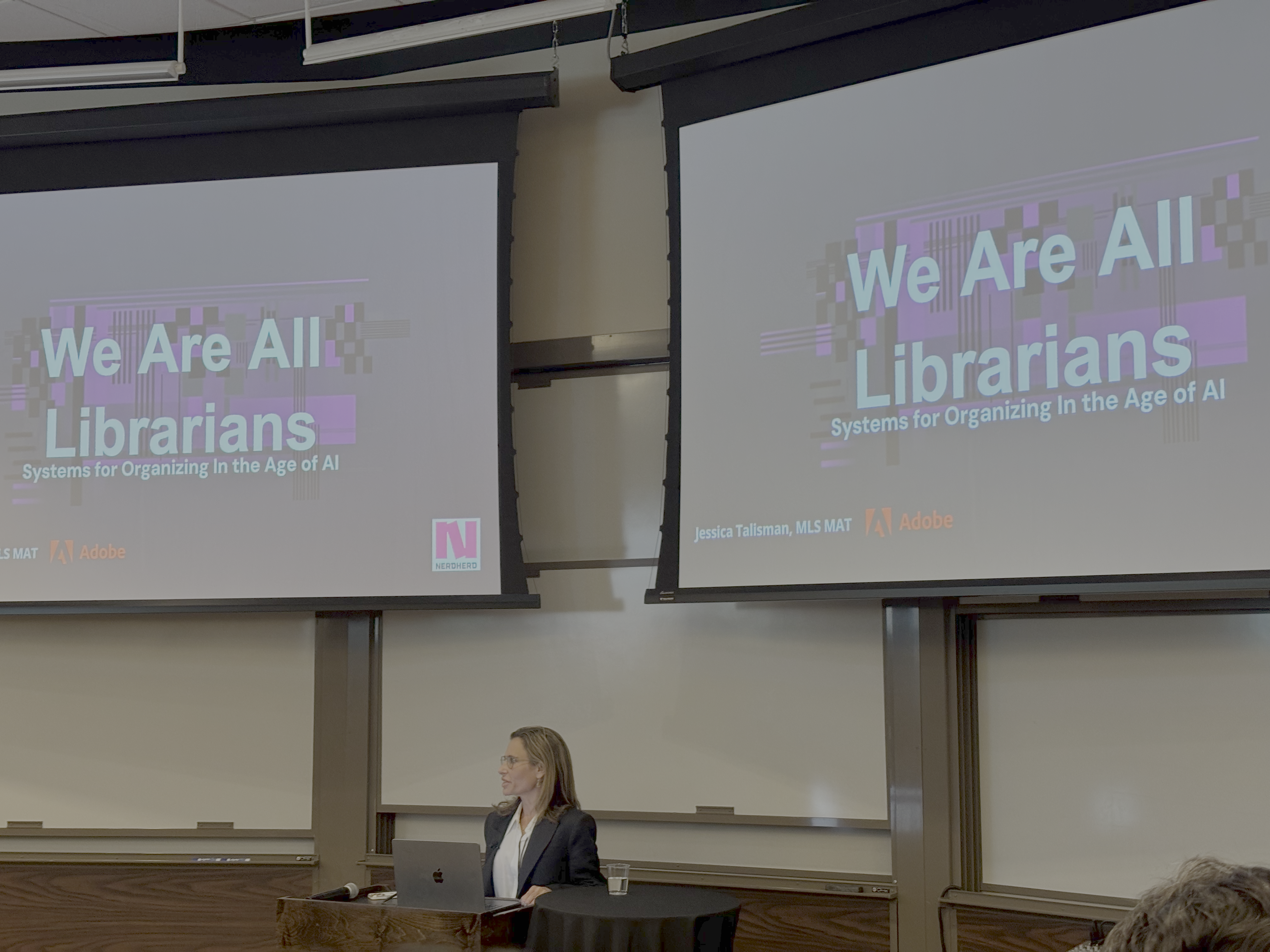
If there was one key takeaway that attendees at Data Day Texas walked away with, it was this: there is a lot that data professionals have to learn from Library & Information Science. Don’t believe me? (I am, after all, quite biased on this.) Ask Joe Reis, Matt Housley, Juan Sequeda, and Tony Baer (podcast episode here). In this Age of AI, context is king, and that context must be machine-readable. It’s common knowledge that your AI systems are only as useful as the data they are trained on, but DDTX attendees learned that the data in your data warehouse - no matter how carefully curated, modeled, and quality tested - is not enough. You also need the context - the knowledge - that often exists only in people’s heads. In addition to Data Engineers, you now need Knowledge Engineers. You’ve heard of “moving up the stack”? Time to also move up the DIKW pyramid, and data is at the bottom. What does it mean for context to be machine readable? Think metadata - and then take it one step further and think of metadata in knowledge graphs. Think of text (in all its myriad forms, from books to emails to human speech), the primary method we have for conveying knowledge. We are re-realizing the importance of data modeling in this Age of AI, so how can we model text data? As Bill Inmon explained, with ontologies and taxonomies. Do you know what field has cultivated and expanded the body of knowledge around metadata, knowledge graphs, ontologies, and taxonomies? Library & Information Sciences. As Tony Baer articulated (article here), the Knowledge Engineer (a role Juan Sequeda introduced in his talk) is an AI-driven evolution of the classical Reference Librarian.
Highlighted Talks:
- Meta Grid - metadata management as an understanding of what already is and embracing it, by Ole Olesen-Bagneux (slides)
- We Are All Librarians: Systems for Organizing in the Age of AI, by Jessica Talisman (slides)
- How to Start Investing in Semantics and Knowledge: A Practical Guide, by Juan Sequeda (summary)
- How to become a hero - the journey to text, by Bill Inmon (book)
- Context Engineering: A Framework for Data Intelligence, by Andrew Nguyen (slides)
“Human” (soft) skills help us connect with the business (and help them)
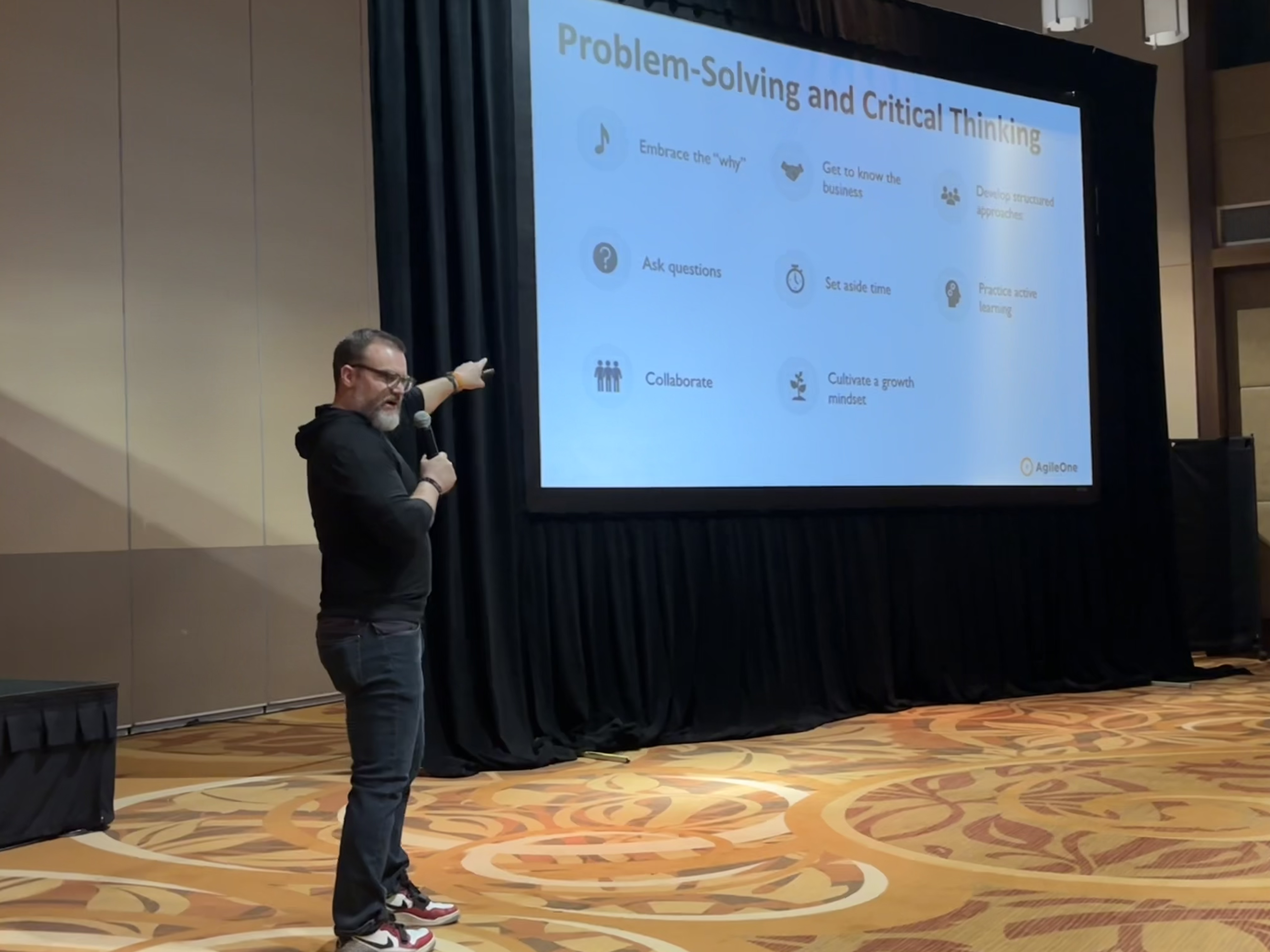
Data teams have spent the last few years struggling to prove ROI and justify their existence on the balance sheet, after costs associated with the “modern data stack” spiraled out of control. We may have fancier tools than ever before, but complaining how the business isn’t “data-driven” enough and throwing data products over the wall for the business to “self-service” isn’t resulting in the ROI we were hoping for. Another takeaway that DDTX attendees walked away with? An acknowledgment that we need to go back to basics and get better at communicating with the business. Yes, talking to people is a skill, and while most people consider it a “soft skill”, it may be among the harder skills you have to learn - but also one of the most important to progressing in your career and growing the impact of your data team. If you don’t want your data team to be treated like an IT service desk - someplace people submit tickets to and expect dashboards in return - but rather a strategic partner that curates the organization’s collective knowledge, then you will need to start valuing “human” skills as much as you do “technical” skills. I’ll note that last year’s opening keynote from Sol Rashidi (“Practitioner turned Executive; what caught me by surprise & lessons I learned about how decisions are really made with data ecosystems”) focused on this takeaway as well, and it is clearly something the data community is still grappling with.
“I’ve learned that people will forget what you said, people will forget what you did, but people will never forget how you made them feel.”
~ Maya Angelou
If you want to communicate and present effectively to executives and key business stakeholders, focus on crafting your story around how you want them to feel, and focus less on specific verbiage. Never underestimate the power of empathy.
Highlighted Talks:
- Bridge Skills: The Hardest Problem Tech Still Can’t Solve, by Eevamaija Virtanen (podcast episode)
- The human side of data: Using technical storytelling to drive action, by Annie Nelson
- Elevating Data in the Business - Bring Data and AI Skills to Life, by Jordan Morrow
- Empowering Change: Building and Sustaining a Data Culture from the Ground Up, by Clair Sullivan (slides)
Data Mesh is a journey, and we’re now in the land of Data Products
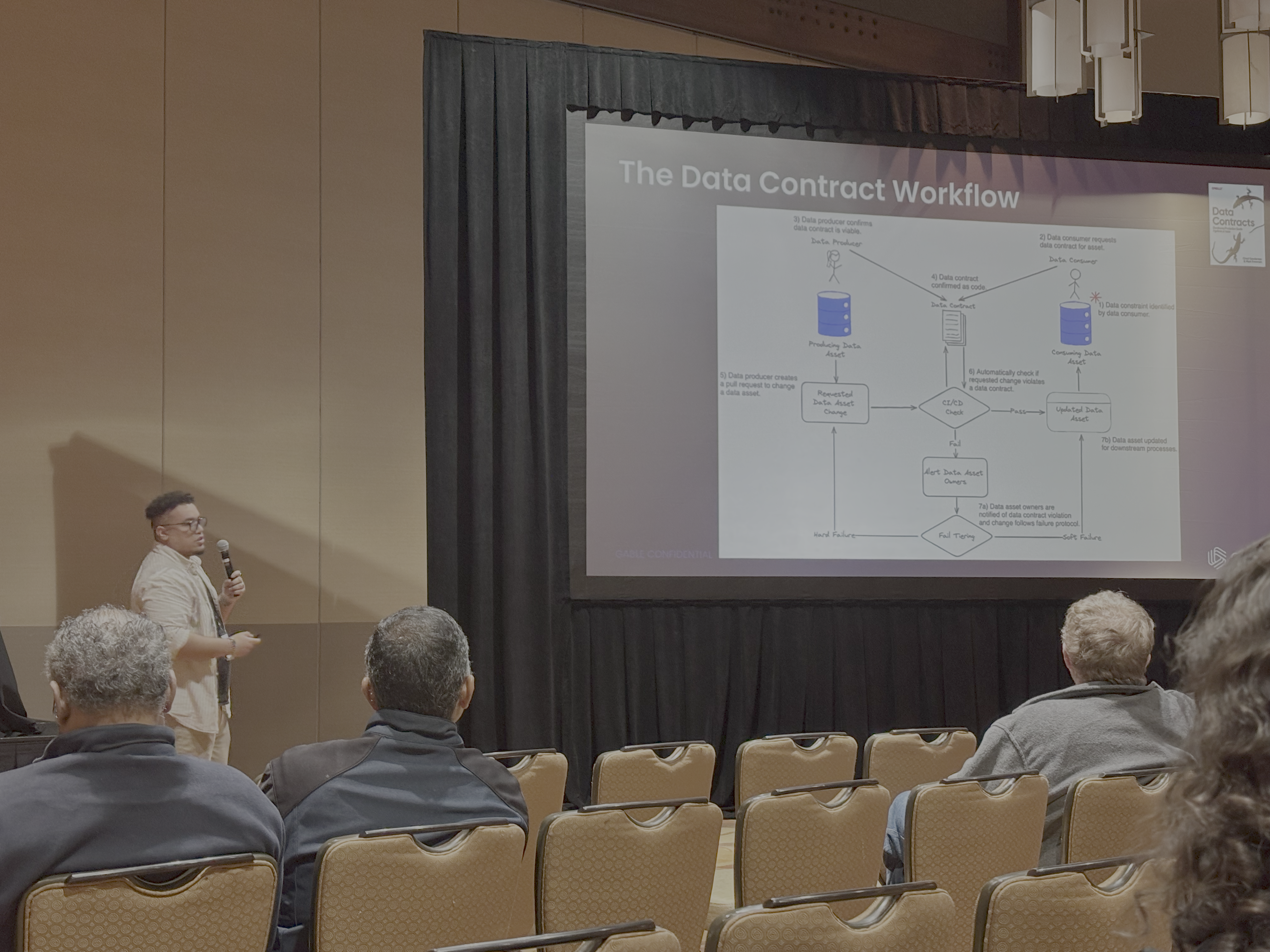
Two years ago Zhamak Dehghani gave the opening keynote for Data Day Texas on Data Mesh, a socio-technical approach to building a decentralized architecture for analytical data. The four pillars of Data Mesh (Domain-oriented ownership, Data as a product, Self-serve data platform, Federated computational governance) are meant to work together and reinforce each other, but when building a Data Mesh from scratch you need to start somewhere. Businesses are already roughly divided into domains (and dotted lines can be drawn until more permanent re-orgs fully align business units with domains), and the next step is to create Data Products (or, treat data as a product). Without Data Products, you can’t really have a self-serve data platform, and there is nothing to govern in a federated computational manner. Data Products seem to be the most visible and lasting legacy of the Data Mesh approach, but I think that is because right now we are in Data Product land (so that is all we see around us). Maybe once we all agree on what a Data Product is and what is needed to make Data Products, we can start seeing actual self-serve data platforms and federated computational governance on the horizon. What does it mean for data to be a product? Zhamak Dehghani has a definition, DJ Patil had the original definition, and perhaps we still don’t know, as JGP gathered us all together on Sunday to discuss and try to arrive at a new definition. One thing is clear, though: we all want to build Data Products, and those doing data engineering and data governance need to start learning how to adopt a product mindset. You don’t need to become a product manager, but you should be able to put on a product manager hat or see what you are building through a product management lens. The first stop through the land of Data Products? Data Contracts - the further upstream the better. Data Contracts should serve as the source of truth for our data’s metadata, and they provide the foundation for any computational data governance implemented further on in our journey.
Highlighted Talks:
- Data Mesh is the Grail, Bitol is your Journey, by Jean-Georges Perrin (summary)
- Introduction to Data Contracts, by Mark Freeman
- Data Governance – It’s Time to Start Over, by Malcolm Hawker
- Escape the Data & AI Death Cycle, Enter the Data & AI Product Mindset, by Anne-Claire Baschet and Yoann Benoit (podcast episode)
- Fundamentals of DataOps, by Lisa Cao
Other themes of note
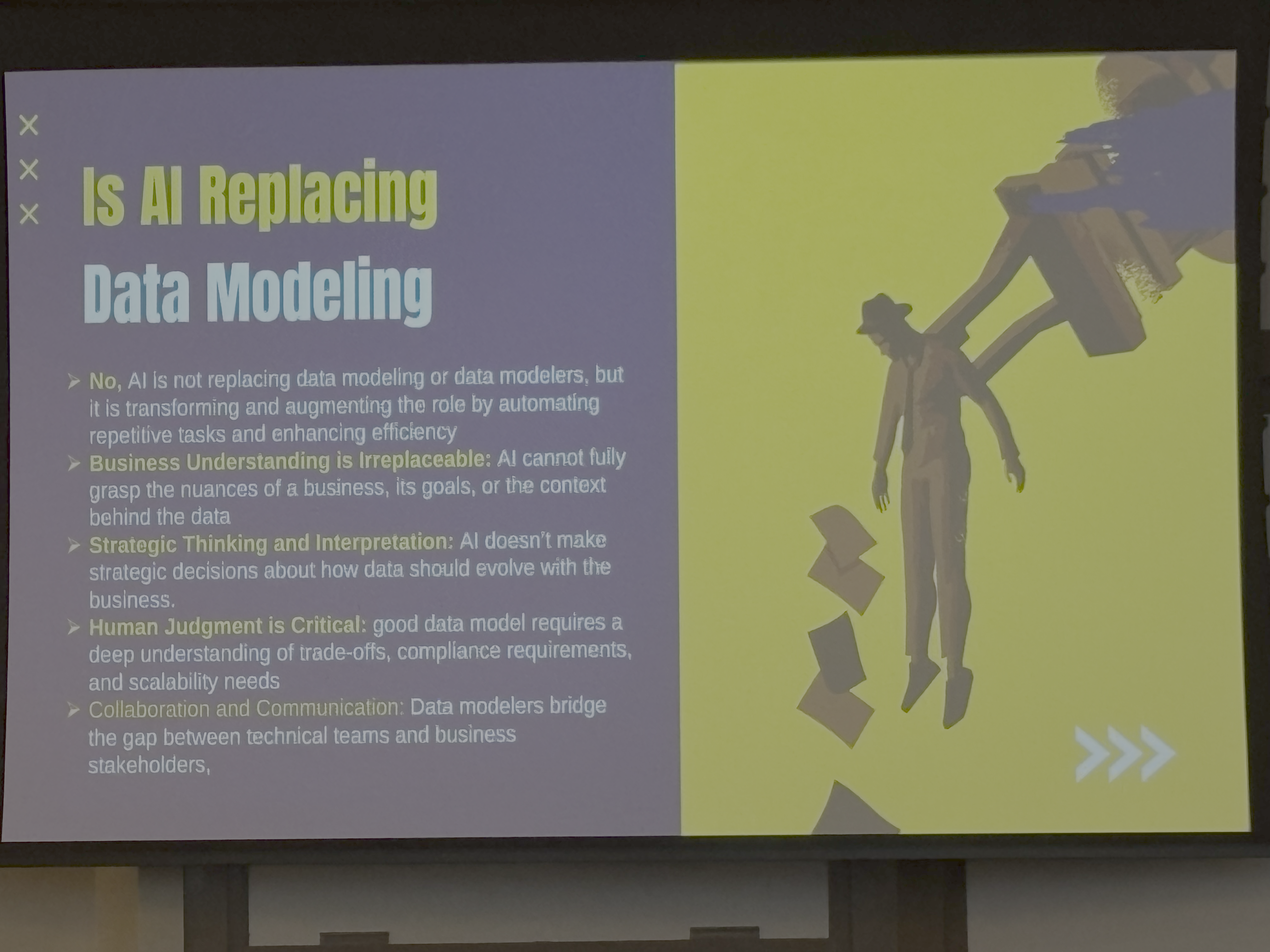
Remember how I said I had to choose just 1 talk of 6 for every time slot? There were a lot of talks I did not get a chance to see. But from what I observed, there are 2 more themes that were prevalent at DDTX: Geeking out over Graphs (of course, this is a tradition at DDTX) and AI in Production (which, not infrequently, overlaps with graphs). A different attendee with different interests may very well say that AI in Production was the most dominant theme, and Geeking out over Graphs was a far more significant theme than those I’ve highlighted, but I can only speak to the talks and discussions I attended, and as much as I may wish I had a time turner (if only for conferences!) … alas, I do not. You can see the full agenda here. I heard a lot of great things about Chip Huyen’s talk, From ML Engineer to AI Engineer, which was heavily influenced by the book she just wrote, AI Engineering. If you missed the talk (like me), you can at least listen to Joe Reis interview Chip about it on his podcast. I also heard some great stories coming out of Michelle Yi’s talk All Your Base Are Belong To Us: Adversarial Attack and Defense and Hala Nelson’s talk Adopting AI in a Large Complex Organization- Aspiration vs Reality. One talk I did catch was Keith Belanger’s on Data Modeling in the Age of AI - the conclusion? LLMs are not going to do your data modeling for you, because data modeling is about understanding business context and thinking strategically. The talk was a spinoff from this article and argued that data modeling (by humans) is more important than ever, and we can take advantage of AI by automating mundane tasks, operating faster and more accurately, allowing us to spend more time focusing on real business value.
Honestly, I could keep going - this is a conference where you could trip and discover a new golden nugget of wisdom. (Side note, if you attended DDTX and have any bootleg recordings of any of the talks, shoot me a DM, because I want to listen to them all). I will say that looking at the agendas for past years, both of these themes show up over and over again, so while the 3 themes I identified may not have been the most dominant (overall), I do think they were the most distinct for this year (compared to previous years).
Diving deeper into my favorite theme: Learnings from Library & Information Science
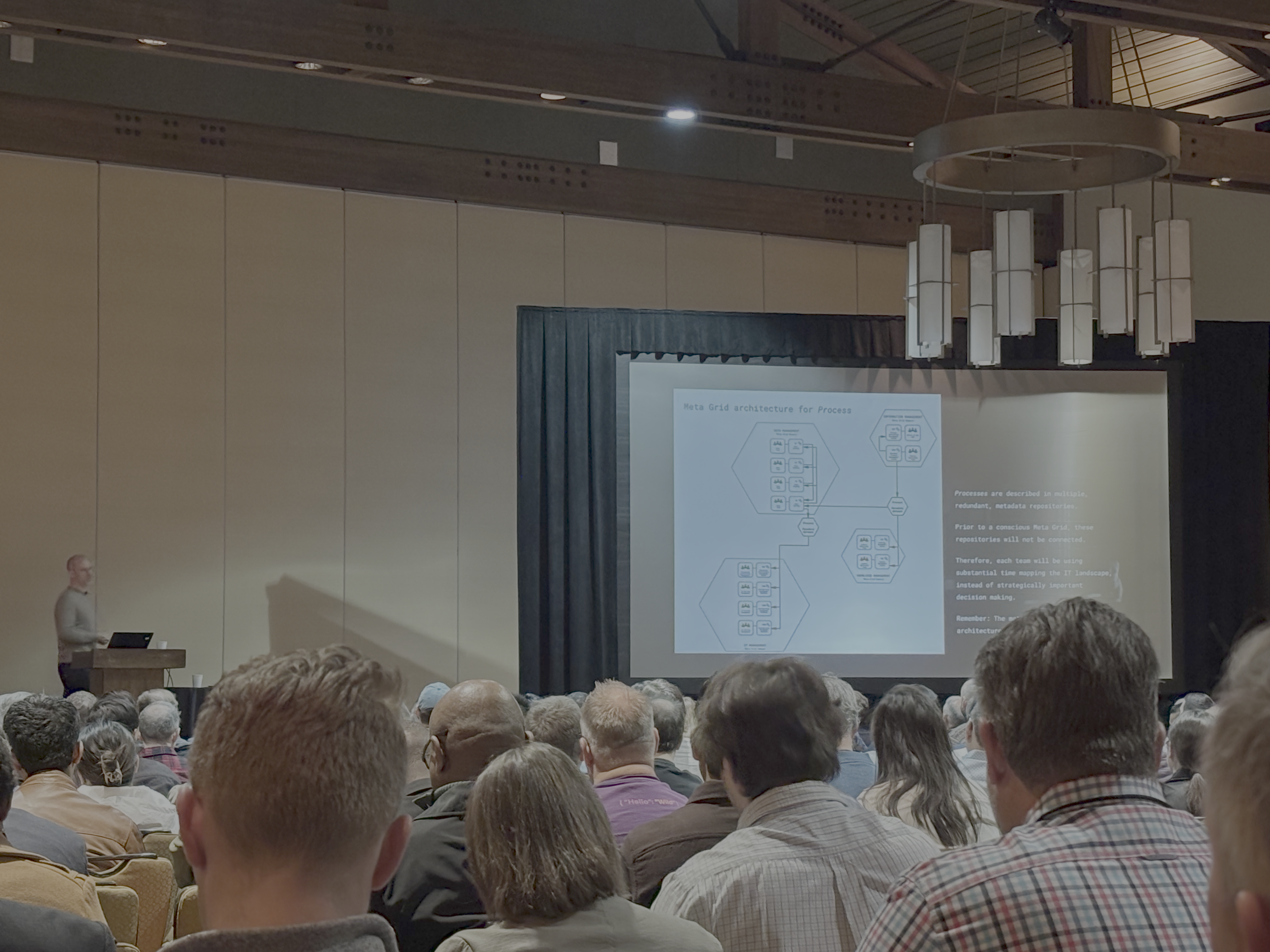
This year’s keynote (the one talk with no competition for your time) was delivered by Ole Olesen-Bagneux, author of The Enterprise Data Catalog and (upcoming) Fundamentals of Metadata Management. Ole has a BA, MA, and Phd in Library & Information Science, and after listening to his talk the entire audience of over 500 data professionals walked away knowing that Library & Information science, libraries, and reference librarians have an important role to play in how we work with data. Ole presented about an architecture for metadata that he has coined the “Meta Grid” (see the slides here), and learnings from Library & Information Science were central to his conception of the Meta Grid. Ole presented the Meta Grid as the 3rd wave of decentralization - the first being microservices, and the 2nd being data mesh. The Meta Grid acknowledges that an organization has many metadata repositories scattered throughout, and proposes that the best solution is not to centralize the metadata into one monolithic catalog, but rather to connect these silo’d and uniquely shaped metadata repositories while maintaining their inherent decentralization. The result? An IT landscape that runs more smoothly, at reduced cost, and is better positioned to ensure data privacy, security, and innovation. If the connection to libraries seems unclear, consider this: the vast majority of a librarian’s work boils down to managing, curating, and navigating metadata, while operating within an network of decentralized yet connected library systems. Ole has spent the past year figuring out how to best articulate and explain the Meta Grid (and writing a book about it) to an audience that at first tries to understand what it is, and then wonders what he is trying to sell. And as Ole reiterated during the keynote, he is not trying to sell or productize anything - he is simply trying to alleviate a deep and enduring pain every organization at large enough scale experiences with their IT landscape, by applying learnings from Library & Information Sciences to corporate metadata management.
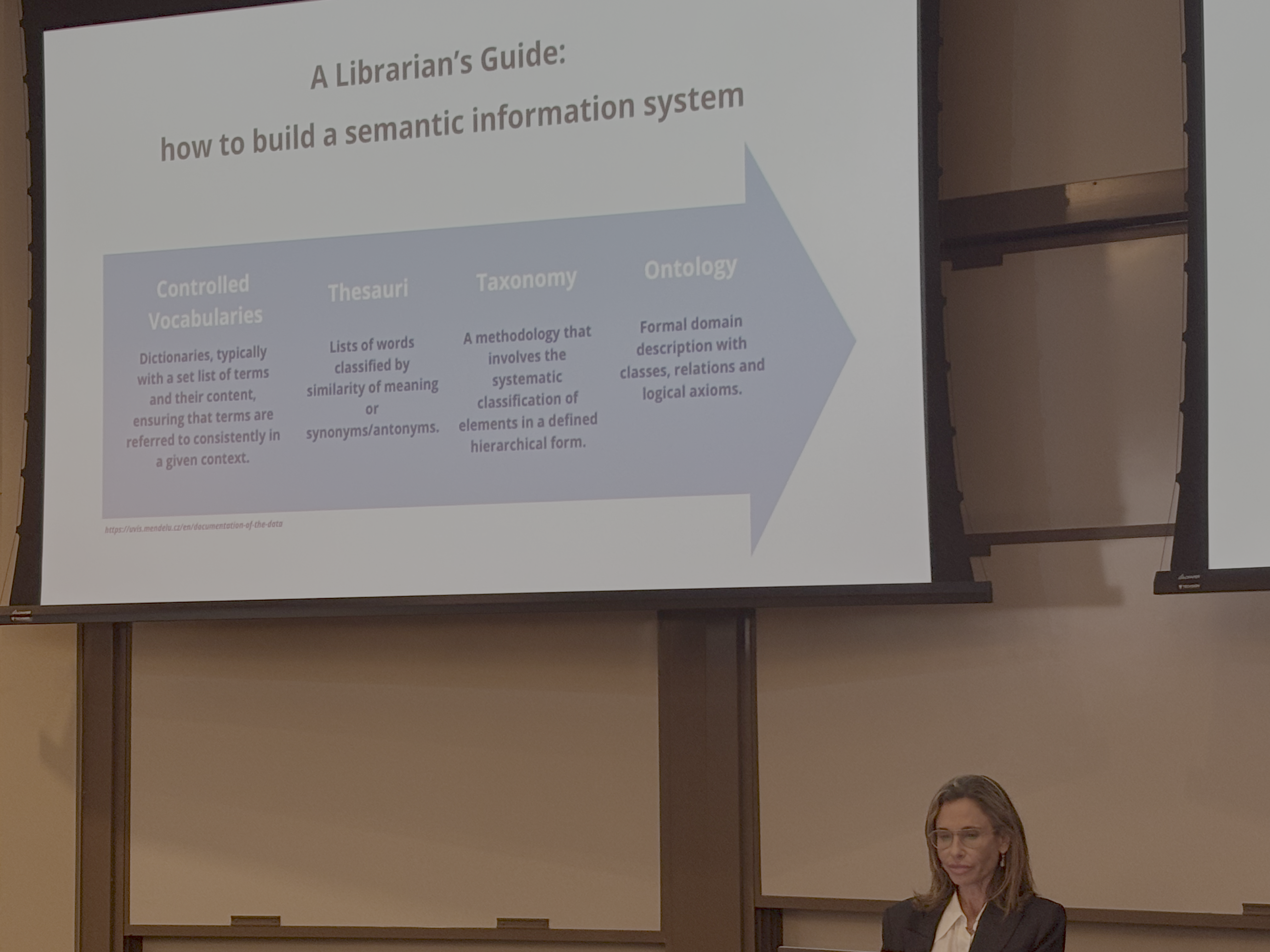
While Ole opened everyone’s mind to the idea that they might have something to learn from Library & Information Science, Jessica Talisman hammered it home with her talk in the afternoon, titled “We Are All Librarians: Systems for Organizing in the Age of AI” (see the slides here). Jessica opened with the argument that if you organize, research, educate, track provenance (lineage), and retrieve information (as most data professionals do), then you are a librarian. And in the age of AI, you would really benefit when performing these librarian activities if you also had some of the tools in a librarian’s toolbox for building a true semantic information system. This is not the first time Jessica has talked about the importance of bringing Library & Information Science skills into AI work - last year she gave a talk titled “What Data Architects and Engineers can learn from Library Science” (recording available here). This year’s talk built on last year’s, and focused in on how to actually approach building an ontology (hint: you don’t start at the ontology step) in a series of steps that Ole suggested afterwards be coined the “Ontology Pipeline”. By starting with a controlled vocabulary, associating those terms in thesauri, then further organizing their relationships in a taxonomy, you will be much better prepared to create the actual final ontology. Jessica highlighted OpenRefine as a tool to mine for themes & terms, and dove into a high-level overview of Linked Data to show the true power of ontologies in an open world (Wikidata being a prime example). She concluded with two pointed citations: (1) that AI systems are cultural technologies (and therefore rely on knowledge management) from Alison Gopnik, and (2) “The Semantic Web is not a separate Web but an extension of the current one, in which information is given well-defined meaning, better enabling computers and people to work in cooperation” from Tim Berners-Lee et al.
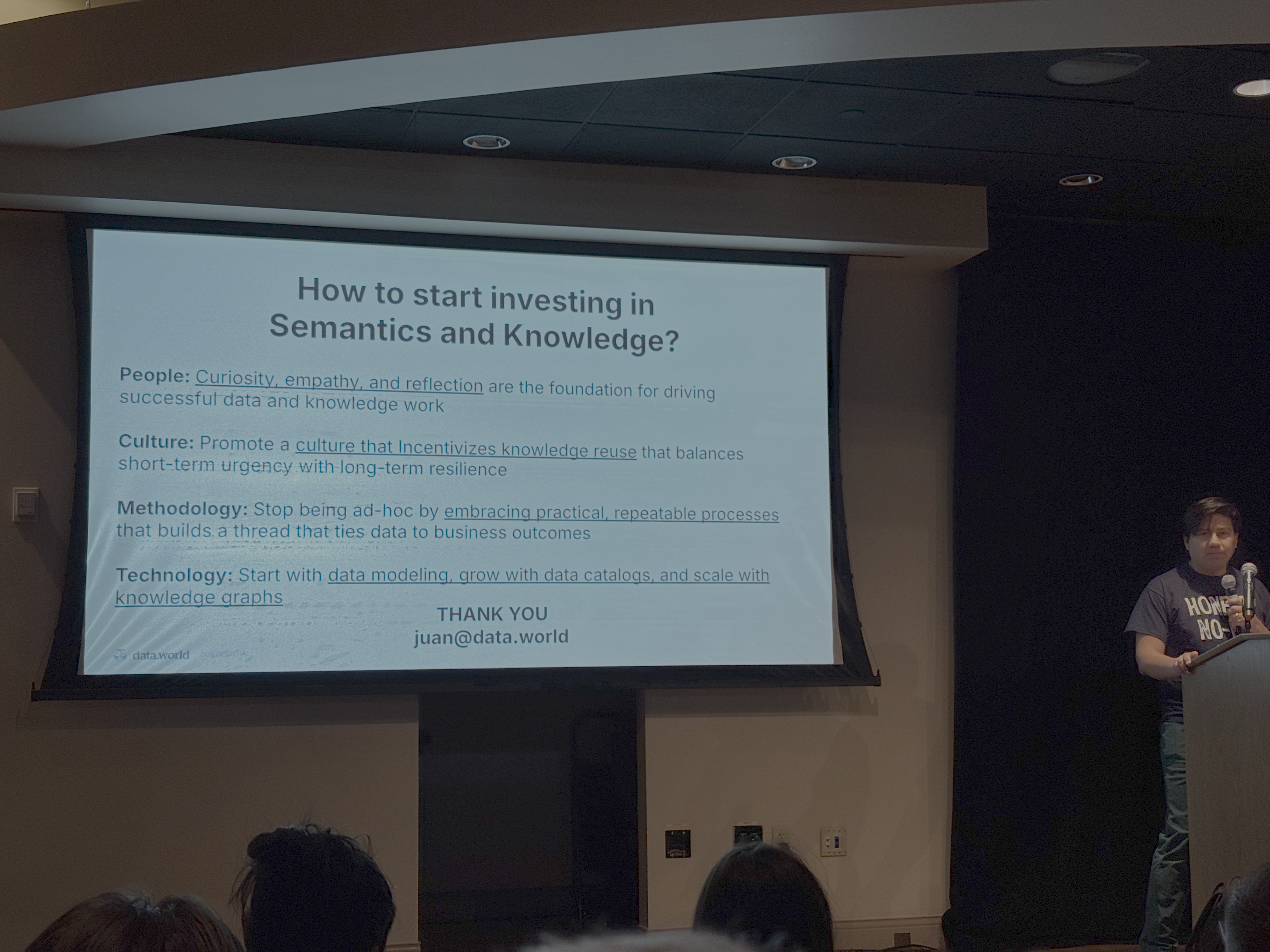
In a very similar vein to Jessica’s talk, Juan Sequeda also focused on the “how” for knowlege management and semantic technologies - only briefly reviewing the “why” (which was the focus of his Coalesce 2024 talk). And much like how Jessica opened her talk by listing out activities many data professionals do and arguing that these are foundational activities for librarians (thus, we are all librarians), Juan listed out many such activities (data cleaning, data transformation, standardization, relation extraction, data governance) and argued that this is socio-technical data & knowledge work (thus, we are all knowledge engineers). He defines semantics as a function that gets applied to data, and results in data with knowledge (or, semantics injects knowledge into data). What is the first step to applying semantics? Be more socratic and start asking why - both on a business and personal level (be empathetic & curious… talk to people!). The highest virtue Juan advocates for is reuse: ad-hoc is the enemy of efficiency, and we should focus on incentivizing the quality & reusability of our data products (and the knowledge that is required to use it!). Two acronyms to remember here: DRY (Don’t Repeat Yourself) and FAIR (Findable, Accessible, Interoperable, Reusable). Juan also highlights a methodology that he first started practicing a decade ago around building knowledge graphs, but is more broadly applicable to “knowledge engineering” work. It has three phases, with a business question as the entry point: Start by capturing the knowledge that the business currently has… then implement it through data modeling/transformation and injecting semantics (possibly via knowledge graphs & ontologies)… and finally make that knowledge accessible through data products and catalogs… but focus this entire process around answering a single business question at a time (then do a 2nd, then a 3rd, and maybe even more questions get answered along the way). With reusability comes another virtue Juan emphasizes: that 1+1 should be >2 (aka economies of scale). Why use knowledge graphs? Because everything is connected, and knowledge graphs are the best way to capture all of those relationships, and surfacing these connections in a data catalog is the ultimate way to maximize the reusability of this knowledge. Much like it is the data engineer’s responsibility to steward data through the data engineering lifecycle, organizations should employ knowledge engineers who are responsible for capturing, implementing, and surfacing knowledge.
Enjoy Reading This Article?
Here are some more articles you might like to read next: